NLP for Document Processing: Transforming Text with AI
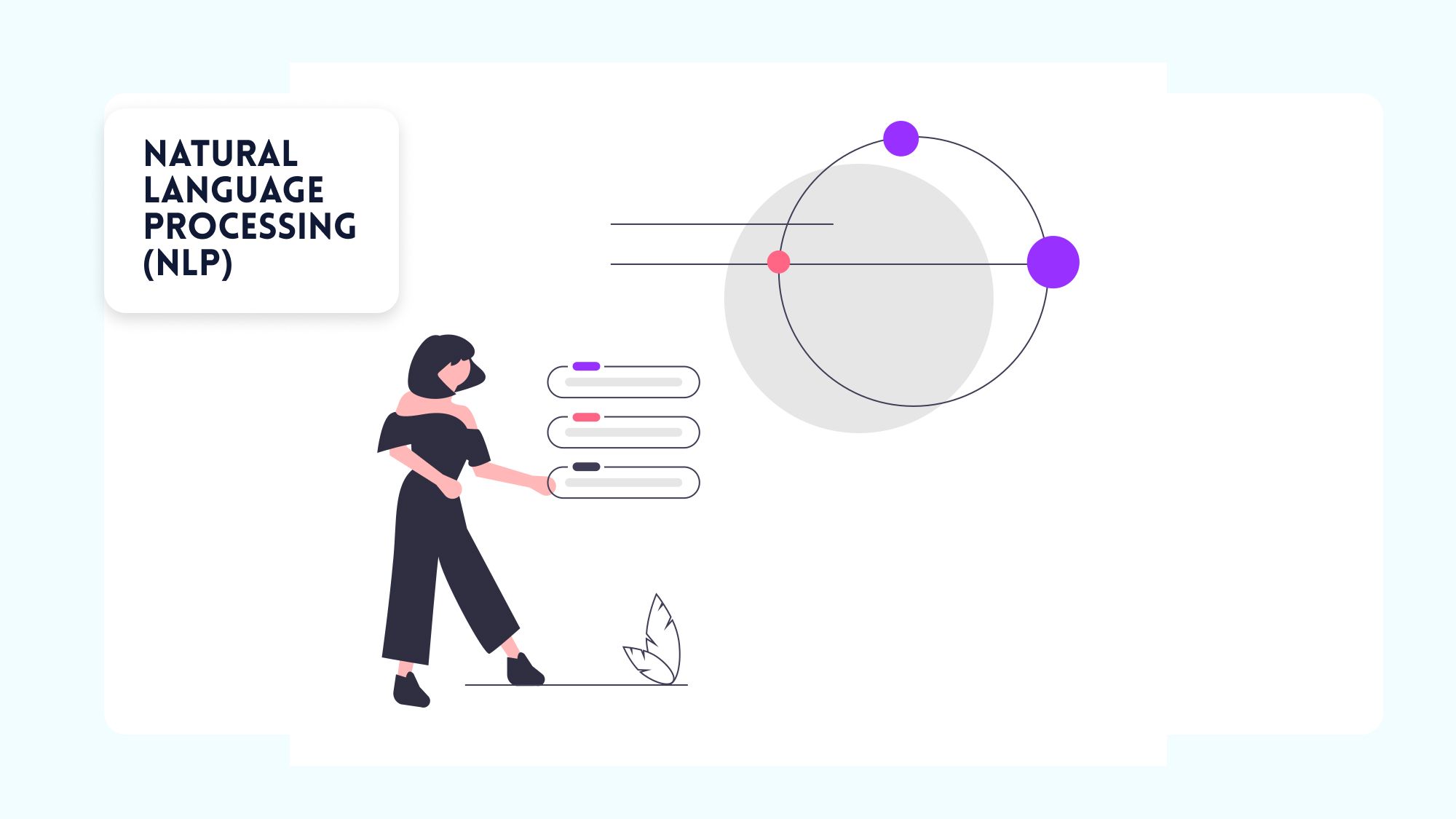
In the era of information overload, the ability to efficiently process and extract meaningful insights from vast amounts of text data is more critical than ever. Natural Language Processing (NLP), a branch of artificial intelligence, has emerged as a powerful tool for document processing, revolutionizing how we handle textual information.
Document processing involves a wide range of tasks, including text classification, named entity recognition, information extraction, summarization, and sentiment analysis. NLP techniques leverage machine learning algorithms to automatically analyze and understand text, enabling organizations to streamline their operations, improve decision-making, and unlock valuable insights buried within documents.
One fundamental task in document processing is text classification, where NLP algorithms automatically assign categories or labels to documents based on their content. For example, an email could be classified as spam or legitimate, a news article could be labeled by topic or customer reviews could be categorized as positive, negative, or neutral. By accurately classifying documents, businesses can automate workflows, prioritize tasks, and efficiently manage large volumes of textual data.
Named Entity Recognition (NER) is another crucial NLP task that identifies and extracts entities such as names of people, organizations, locations, or dates from documents. This process enables businesses to identify key information in unstructured text and organize it into structured formats. NER finds applications in various domains, including information retrieval, knowledge graph construction, and customer relationship management.
Information extraction goes beyond named entities and aims to identify specific facts and relationships within documents. By employing techniques like parsing, pattern matching, and machine learning, NLP algorithms can extract structured information from unstructured text. For example, in the context of legal documents, information extraction can automatically identify clauses, contract terms, or obligations, saving time and effort in manual review processes.
Summarization is another critical document processing task that involves condensing lengthy texts into shorter versions while retaining their core meaning. Automatic summarization can be extractive, where key sentences are selected and stitched together, or abstractive, where the system generates new sentences to summarize the content. Summarization has applications in various domains, including news articles, legal documents, and academic research papers, enabling users to quickly grasp the main points without reading the entire text.
Sentiment analysis, also known as opinion mining, involves determining the sentiment expressed in a given piece of text, whether it is positive, negative, or neutral. This task is particularly relevant for customer feedback analysis, brand monitoring, and social media sentiment tracking. Sentiment analysis allows organizations to understand public opinion, identify emerging trends, and respond promptly to customer needs or concerns.
To achieve these document processing tasks, NLP techniques heavily rely on the availability of high-quality training data. Large-scale annotated datasets are used to train machine learning models, enabling them to generalize and make accurate predictions on unseen data. Additionally, advancements in deep learning, particularly with transformer models like BERT and GPT, have significantly improved the performance of NLP tasks, pushing the boundaries of what is possible in document processing.
In conclusion, NLP has revolutionized document processing by automating tasks that were traditionally time-consuming and resource-intensive. By leveraging NLP techniques, businesses can extract valuable insights from large volumes of text, improve operational efficiency, and make data-driven decisions. As NLP continues to evolve, we can expect further advancements in document processing, enabling us to unlock even more value from textual information in the future.